Unlocking Success with Data Annotation in Machine Learning
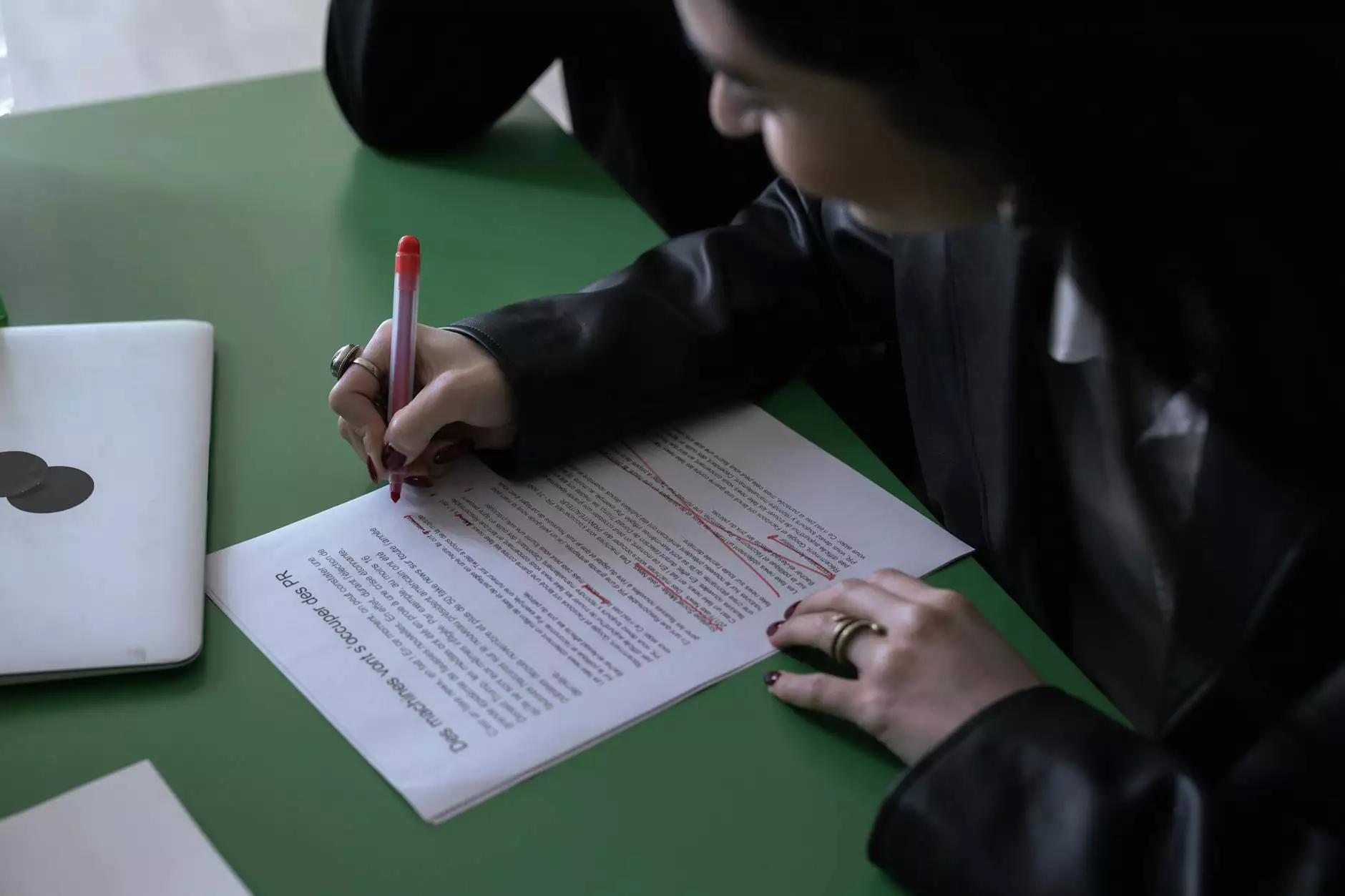
In the rapidly evolving digital landscape, businesses are continuously seeking innovative solutions to enhance their operations and improve customer experiences. At the heart of such innovation lies machine learning, a technology that is transforming various industries by automating processes and generating valuable insights. However, the effectiveness of machine learning algorithms significantly hinges on one crucial element: data annotation.
What is Data Annotation?
Data annotation refers to the process of labeling data to provide context. In the realm of machine learning, models learn from this labeled data to make informed decisions or predictions. Imagine teaching a child to recognize animals; you would show them various pictures of animals alongside their names. Similarly, data annotation equips algorithms with examples that help them understand and learn patterns. This is especially pivotal for fields that rely heavily on visual identification, such as artificial intelligence (AI), natural language processing (NLP), and voice recognition.
The Significance of Data Annotation in Machine Learning
Properly annotated data is essential for creating reliable and accurate machine learning models. The significance of data annotation in machine learning can be broken down into several key points:
- Enhanced Model Accuracy: Accurate and well-labeled data leads to better predictions and insights from machine learning models.
- Facilitation of Supervised Learning: Supervised learning, a common approach in machine learning, relies heavily on annotated datasets to train algorithms effectively.
- Improved Data Quality: A robust annotation process improves the overall quality of the dataset, making it easier for algorithms to derive meaningful insights.
- Cost-Effectiveness: While investing in quality data annotation may seem daunting, the long-term benefits of improved model performance significantly outweigh the initial costs.
Types of Data Annotation
Data annotation comes in various forms, catering to the unique needs of different machine learning projects. Here are some common types:
1. Image Annotation
This involves labeling images with tags or segments. Image annotation is widely used in computer vision tasks. Common techniques include:
- Bounding Boxes: Drawing rectangles around objects of interest in an image.
- Polygon Annotation: Enclosing irregularly shaped objects with a polygon.
- Semantic Segmentation: Assigning a class label to each pixel in the image for detailed analysis.
2. Text Annotation
Text annotation involves marking up text data with labels. This is critical for NLP applications. Examples include:
- Named Entity Recognition (NER): Identifying and classifying key entities in text, such as names, dates, and locations.
- Sentiment Analysis: Labeling expressions as positive, negative, or neutral to understand customer sentiments.
3. Audio Annotation
Audio annotation involves transcribing spoken language into text or tagging audio clips for different sounds or tones. This is particularly useful for:
- Speech Recognition: Converting spoken words into text.
- Emotion Recognition: Analyzing tone and pitch for mood detection.
The Process of Data Annotation
To effectively implement data annotation, businesses should follow a structured process:
- Data Collection: Gather raw data that needs to be annotated. This could be images, text documents, audio files, etc.
- Annotation Strategy: Develop a clear annotation strategy outlining guidelines for labeling the data.
- Annotation Tool Selection: Choose appropriate tools and software that streamline the annotation process.
- Execution: Carry out the annotation task, ensuring accuracy at every step.
- Quality Assurance: Review the annotated data for consistency and accuracy, correcting any mistakes as necessary.
Why Choose KeyMakr for Your Data Annotation Needs?
As businesses explore the potential of machine learning, the need for quality data annotation becomes paramount. Here’s why you should consider KeyMakr for your data annotation requirements:
1. Expertise in Various Industries
KeyMakr has experience working across numerous industries, from home services to automotive, which allows us to understand the unique challenges and requirements of different sectors.
2. Advanced Annotation Tools
We utilize state-of-the-art annotation tools that ensure high precision and efficiency in the annotation process.
3. Customizable Solutions
Our services are tailored to meet your specific needs, whether you require large volumes of labeled data or specialized annotation techniques.
4. Stringent Quality Control
At KeyMakr, quality is our priority. We implement rigorous quality control measures to ensure that your annotated data is both accurate and reliable.
5. Timely Delivery
We understand the value of time in business. Our team is committed to delivering quality annotated datasets within agreed timelines.
Benefits of Data Annotation for Businesses
Investing in data annotation can provide businesses with a multitude of benefits:
- Improved Decision Making: With high-quality, labeled data, businesses can derive actionable insights that facilitate informed decision-making.
- Increased Competitive Edge: Businesses that leverage machine learning effectively can outperform competitors who fail to adopt these technologies.
- Enhanced Customer Experience: By analyzing customer interactions through annotated data, businesses can anticipate needs and enhance service quality.
- Automation of Tasks: Well-trained models can automate tasks, reduce human error, and free up resources for strategic priorities.
Emerging Trends in Data Annotation
As technology advances, the domain of data annotation is also evolving. Some emerging trends include:
1. Crowdsourced Annotation
Utilizing crowdsourcing allows businesses to tap into a larger pool of annotators, making the data annotation process quicker and often cheaper without sacrificing quality.
2. AI-Assisted Annotation
AI tools are now being employed to assist human annotators by automating parts of the annotation process, which drastically reduces the time and cost involved.
3. Real-Time Annotation
As machine learning models are deployed in real-time scenarios, there is a growing need for real-time data annotation to deal with live data inputs efficiently.
Conclusion
In conclusion, data annotation in machine learning is an indispensable component that drives the success of AI projects. As businesses like KeyMakr take on the responsibility of annotating data with precision, companies can focus more on leveraging innovative solutions to stay ahead in their industries. Whether you're in the domain of home services or locksmith services, adopting proper data annotation techniques will empower your machine learning initiatives, resulting in enhanced operational efficiency and superior customer satisfaction.
Ready to take your machine learning projects to new heights? Trust in KeyMakr's expertise in data annotation to provide you with the quality data that is essential for your success! Contact us today and let's embark on this journey towards innovation together.
data annotation machine learning